R&D
Exploring the future of streaming recommendations.
March 13, 2024
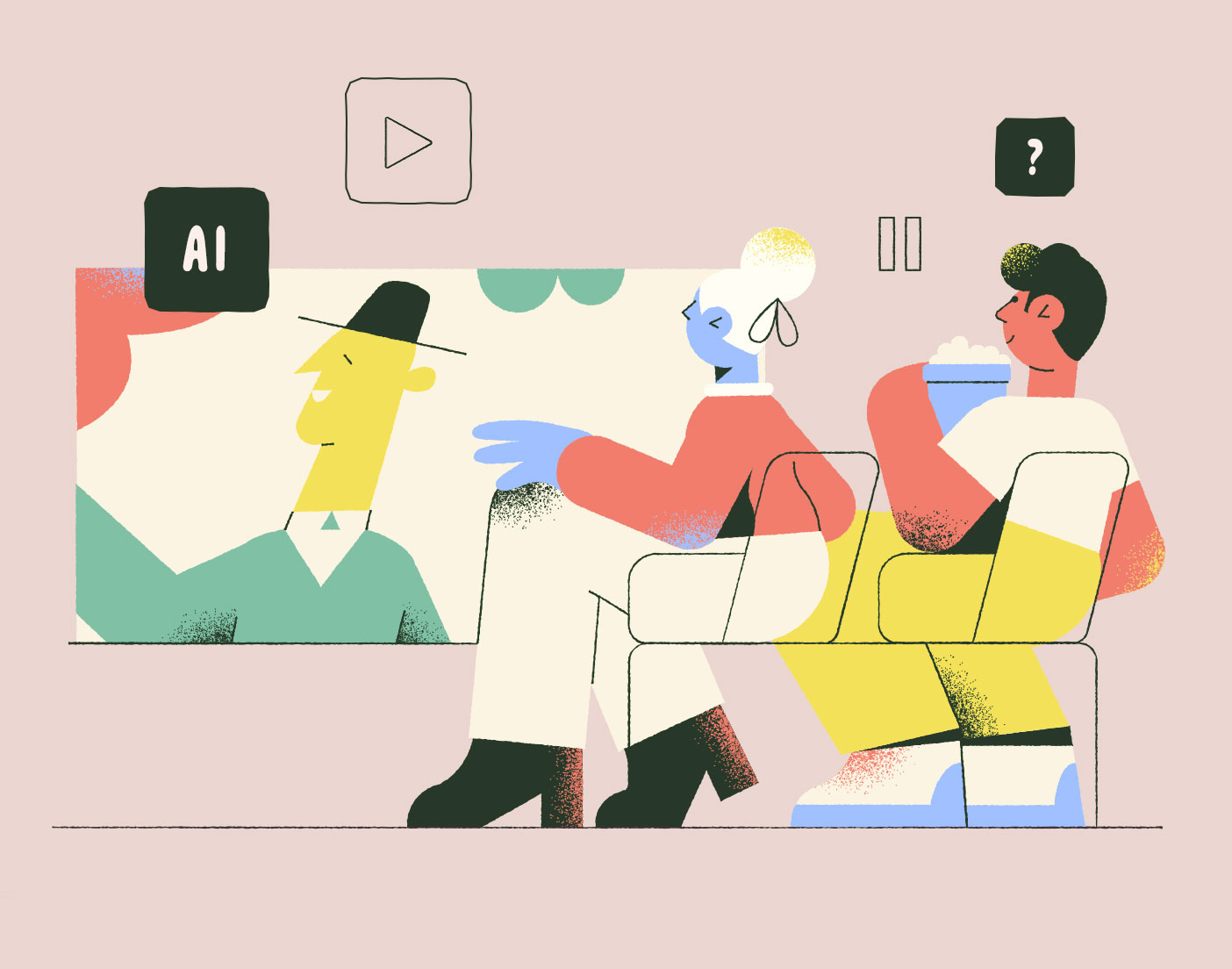
Long story short
- Users are often puzzled by algorithmic content recommendations.
- REDspace is researching transparent, explainable, and personalized recommendations.
- Explainable AI analyzes a user’s preferences, and their interactions with videos.
- Benefits: User empowerment, trust-building, enhanced experience.
- REDspace's goal: Revolutionize streaming recommendations, demystify processes.
Why am I being recommended this?
In a world with instant access to never-ending entertainment and media content, platforms like YouTube, Spotify, and Netflix have made recommendation systems a part of our daily lives. But the mysterious nature of these algorithms often leaves users in the dark, leaving you asking the question, “Why am I being recommended this?”
Traditionally, recommendation systems utilize user data—their history, interaction logs, and behavior—to train and refine AI models for personalized recommendations. For instance, Spotify automatically generates a playlist based on the user’s activities. Netflix shows the similarity between recommended items as a percentage or changes the arrangement of items in a row. In both cases, the results do not explain why the recommendations and adjustments happened. This lack of explicit explanation of how these systems generate recommendations makes it hard for users to understand the algorithm at work.
A look behind-the-scenes.
At REDspace, we aim to explore the role of transparency in shaping the success and effectiveness of recommendation systems. Data Scientist Reza Rajabi elaborates on the challenges posed by existing systems, “Users are often not told why certain recommendations are made. It’s a critical gap we want to bridge.”
The personalized and explainable recommendation system we’ve been working on addresses this gap. Rajabi explains, “We analyze user preferences and behaviors, using video attributes like metadata, tags, and content to identify crucial factors in recommendations. And by applying modern content-based algorithms and collaborative filtering models in our system we can identify important factors in recommendations.” Unlike conventional systems, we take various explainability approaches, including SHAP (SHapley Additive exPlanations), to offer users comprehensive and accurate insights into why specific content is presented to them. We want to move beyond generic suggestions, helping users comprehend and connect with recommendations on a personal level.
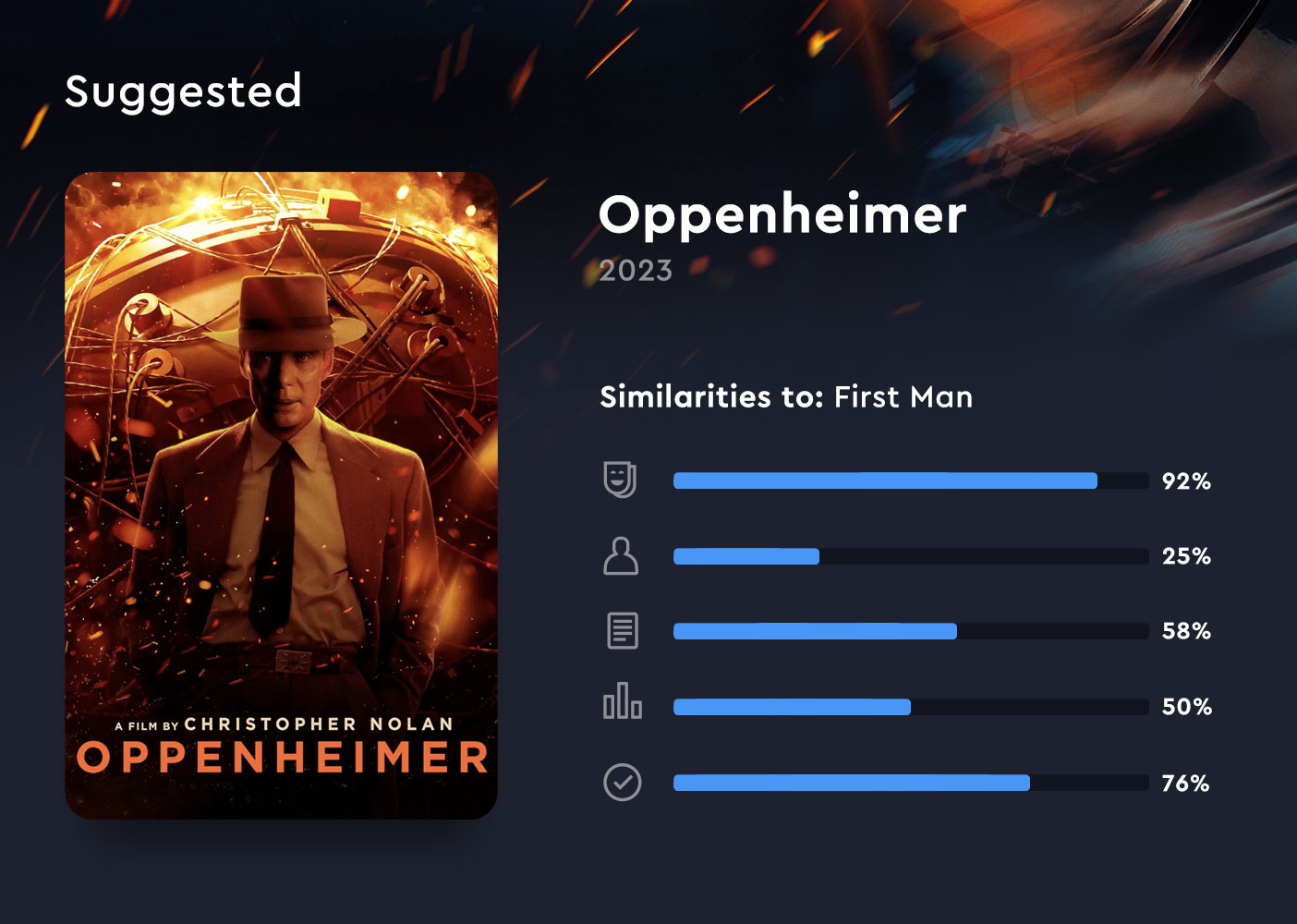
One of the many ways an app could display the data it has behind its recommendations. In this case, a user is shown the similarities between two films in genre, cast, story, info, and the user’s previous actions with other films.
Why should you care?
As we see a shift in the understanding of user habits and preferences, the role of Explainable AI could be a crucial element in the future of streaming. Transparency doesn’t just address current challenges but may also lay the foundation for adapting to evolving user behaviors down the road.
Some benefits of using Explainable AI for recommendation systems might include:
Empowering users
Knowing why particular content is recommended gives users a sense of control over their media consumption. It helps them to make informed decisions and engage with a platform based on their preferences.
Building trust
If users understand where recommendations come from, they’re more likely to trust suggestions.
Improving the user experience
Users can navigate a platform quicker, finding content that actually aligns with their interests. There’s also the potential for users to give feedback to the AI in real time with things like added filtering—giving them even deeper customization and control.
Find a deeper understanding of your users.
REDspace leverages AI-based collaborative filtering approaches (e.g., neural-network-based algorithms) to showcase the most relevant results to users. By generating recommendations based on both user and video attributes and their interactions, we ensure a more tailored and engaging experience.
Our goal is not just to revolutionize streaming recommendations; we want to demystify the processes.
Personalization is further improved through the use of the Matrix Factorization (MF) model, a linear algebra technique. Rajabi adds,“This model helps discover latent features underlying the interactions between users and videos. These features give a more compact representation of user tastes and video descriptions.”
Essentially, our goal is not just to revolutionize streaming recommendations; we want to demystify the processes, giving users more power with insights into the “why” behind their personalized content recommendations.
Want to help shape the future of streaming recommendations?
We value your insights! If you’re an AI enthusiast, someone passionate about personalized experiences, or looking to improve your streaming platform, we want to hear from you. Contact us to share your thoughts on streaming recommendations or suggest features you’d like us to look at.
We’re looking for new ways to make your life easier with Artificial Intelligence.
If you like this article, you might be interested in learning more about some of the other research we’ve done in the world of AI.